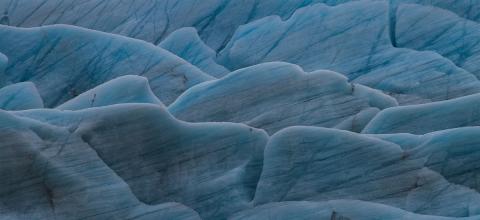
GPU-accelerated Ice Sheet Flow Modeling
Sea levels are rising (3.7 mm/year and increasing!)! The primary contributor to rising sea levels is enhanced polar ice discharge due to climate change. However, their dynamic response to climate change remains a fundamental uncertainty in future projections. Computational cost limits the simulation time on which models can run to narrow the uncertainty in future sea level rise predictions. The project's overarching goal is to leverage GPU hardware capabilities to significantly alleviate the computational cost and narrow the uncertainty in future sea level rise predictions. Solving time-independent stress balance equations to predict ice velocity or flow is the most computationally expensive part of ice-sheet simulations in terms of computer memory and execution time. The PI developed a preliminary ice-sheet flow GPU implementation for real-world glaciers. This project aims to investigate the GPU implementation further, identify bottlenecks and implement changes to justify it in the price to performance metrics to a "standard" CPU implementation. In addition, develop a performance portable hardware (or architecture) agnostic implementation.