ACCESS MATCH Services connects researchers with experts to help you select the right system, run on a supercomputer, and solve basic code and research problems.
MATCH Plus



We will match you with an expert based on your needs.
Solve research needs like expanding code, transitioning from lab computers to HPC or introducing new techniques.
Steps in a MATCH Plus Engagement
1
An ACCESS community member identifies a need for support and submits an engagement request.
2
MATCH staff will work with you to scope the engagement and match you with an appropriate expert.
3
The MATCH Plus expert works with you to address the research need.
4
When the goal is achieved notes are logged to benefit the community.
MATCH Plus FAQ
How can I find out if my project is a good fit for a MATCH engagement?
What’s the timeline for a MATCH Plus engagement?
Is there a cost for using MATCH Plus?
MATCH Engagements
Find out about the ACCESS MATCH Engagements and if you want to get involved use the “I’m interested” button on recruiting engagements.
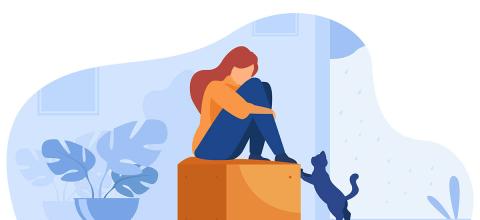
Investigation of robustness of state of the art methods for anxiety detection in real-world conditions
<p>I am new to ACCESS. I have a little bit of past experience running code on NCSA's Blue Waters. As a self-taught programmer, it would be interesting to learn from an experienced mentor. </p><p>Here's an overview of my project:</p><p>Anxiety detection is topic that is actively studied but struggles to generalize and perform outside of controlled lab environments. I propose to critically analyze state of the art detection methods to quantitatively quantify failure modes of existing applied machine learning models and introduce methods to robustify real-world challenges. The aim is to start the study by performing sensitivity analysis of existing best-performing models, then testing existing hypothesis of real-world failure of these models. We predict that this will lead us to understand more deeply why models fail and use explainability to design better in-lab experimental protocols and machine learning models that can perform better in real-world scenarios. Findings will dictate future directions that may include improving personalized health detection, careful design of experimental protocols that empower transfer learning to expand on existing reach of anxiety detection models, use explainability techniques to inform better sensing methods and hardware, and other interesting future directions.</p>
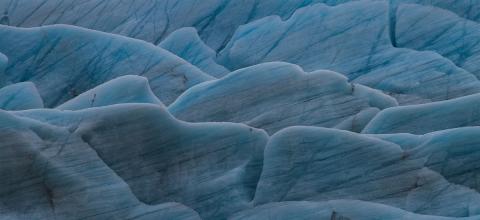
GPU-accelerated Ice Sheet Flow Modeling
<p>Sea levels are rising (3.7 mm/year and increasing!)! The primary contributor to rising sea levels is enhanced polar ice discharge due to climate change. However, their dynamic response to climate change remains a fundamental uncertainty in future projections. Computational cost limits the simulation time on which models can run to narrow the uncertainty in future sea level rise predictions. The project's overarching goal is to leverage GPU hardware capabilities to significantly alleviate the computational cost and narrow the uncertainty in future sea level rise predictions. Solving time-independent stress balance equations to predict ice velocity or flow is the most computationally expensive part of ice-sheet simulations in terms of computer memory and execution time. The PI developed a preliminary ice-sheet flow GPU implementation for real-world glaciers. This project aims to investigate the GPU implementation further, identify bottlenecks and implement changes to justify it in the price to performance metrics to a "standard" CPU implementation. In addition, develop a performance portable hardware (or architecture) agnostic implementation.</p>
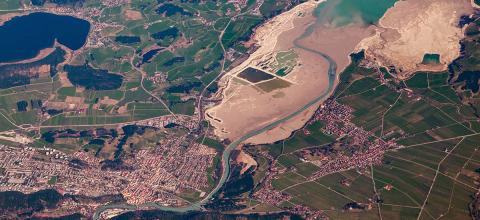
Adapting a GEOspatial Agent-based model for Covid Transmission (GeoACT) for general use
<p>GeoACT (GEOspatial Agent-based model for Covid Transmission) is a designed to simulate a range of intervention scenarios to help schools evaluate their COVID-19 plans to prevent super-spreader events and outbreaks. It consists of several modules, which compute infection risks in classrooms and on school buses, given specific classroom layouts, student population, and school activities. The first version of the model was deployed on the Expanse (and earlier, COMET) resource at SDSC and accessed via the Apache Airavata portal (geoact.org). The second version is a rewrite of the model which makes it easier to adjust to new strains, vaccines and boosters, and include detailed user-defined school schedules, school floor plans, and local community transmission rates. This version is nearing completion. We’ll use Expanse to run additional scenarios using the enhanced model and the newly added meta-analysis module. The current goal is to make the model more general so that it can be used for other health emergencies. GeoACT has been in the news, e.g. <a href="https://ucsdnews.ucsd.edu/feature/uc-san-diego-data-science-undergrads-… San Diego Data Science Undergrads Help Keep K-12 Students COVID-Safe</a>, and <a href="https://www.hpcwire.com/2022/01/13/sdsc-supercomputers-helped-enable-sa… Supercomputers Helped Enable Safer School Reopenings</a> (HPCWire 2022 Editors' Choice Award)</p>