Affinity Groups
Logo | Name | Description | Tags | Join |
---|---|---|---|---|
Launch | Launch is a regional computational resource that supports researchers incorporating computational and data-enabled approaches in their scientific workflows at eleven under-resourced institutions in… | aidata-analysis | Login to join |
Announcements
Title | Date |
---|---|
CI Pathways: Leading the Way to Effective CI Use | 02/20/25 |
NSF requests research and education use cases for NAIRR | 02/22/24 |
Upcoming Events & Trainings
Title | Date |
---|---|
Getting Started with Jetstream2 | 3/06/25 |
Getting Started with Jetstream2 | 3/06/25 |
Getting Started with Jetstream2 | 3/06/25 |
Topics from Ask.CI
Knowledge Base Resources
Title | Category | Tags | Skill Level |
---|---|---|---|
AI Institutes Cyberinfrastructure Documents: SAIL Meeting | Learning | access-accountaidata-analysis +1 more tags | Beginner, Intermediate, Advanced |
An Introduction to the Julia Programming Language | Learning | aidata-analysismachine-learning +1 more tags | Beginner |
Applications of Machine Learning in Engineering and Parameter Tuning Tutorial | Learning | data-analysismachine-learningpython | Beginner, Intermediate |
Engagements
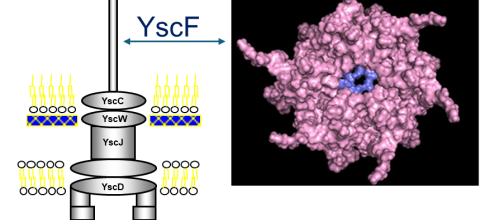
Prediction of Polymerization of the Yersinia Pestis Type III Secretion System
Yersinia pestis, the bacterium that causes the bubonic plague, uses a type III secretion system (T3SS) to inject toxins into host cells. The structure of the Y. pestis T3SS needle has not been modeled using AI or cryo-EM. T3SS in homologous bacteria have been solved using cryo-EM. Previously, we created possible hexamers of the Y. pestis T3SS needle protein, YscF, using CollabFold and AlphaFold2 Colab on Google Colab in an effort to understand more about the needle structure and calcium regulation of secretion. Hexamers and mutated hexamers were designed using data from a wet lab experiment by Torruellas et. al (2005). T3SS structures in homologous organisms show a 22 or 23mer structure where the rings of hexamers interlocked in layers. When folding was attempted with more than six monomers, we observed larger single rings of monomers. This revealed the inaccuracies of these online systems. To create a more accurate complete needle structure, a different computer software capable of creating a helical polymerized needle is required. The number of atoms in the predicted final needle is very high and more than our computational infrastructure can handle. For that reason, we need the computational resources of a supercomputer. We have hypothesized two ways to direct the folding that have the potential to result in a more accurate needle structure. The first option involves fusing the current hexamer structure into one protein chain, so that the software recognizes the hexamer as one protein. This will make it easier to connect multiple hexamers together. Alternatively, or additionally the cryo-EM structures of the T3SS of Shigella flexneri and Salmonella enterica Typhimurium can be used as models to guide the construction of the Y. pestis T3SS needle. The full AlphaFold library or a program like RoseTTAFold could help us predict protein-protein interactions more accurately for large structures. Based on our needs we have identified the TAMU ACES, Rockfish and Stampede-2 as promising resources for this project. The generated model of the Y. pestis T3SS YscF needle will provide insight into a possible structure of the needle.
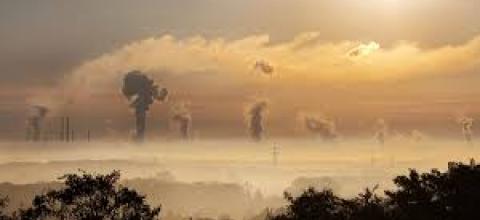
Bayesian nonparametric ensemble air quality model predictions at high spatio-temporal daily nationwide 1 km grid cell
I aim to run a Bayesian Nonparametric Ensemble (BNE) machine learning model implemented in MATLAB. Previously, I successfully tested the model on Columbia's HPC GPU cluster using SLURM. I have since enabled MATLAB parallel computing and enhanced my script with additional lines of code for optimized execution.
I want to leverage ACCESS Accelerate allocations to run this model at scale.
The BNE framework is an innovative ensemble modeling approach designed for high-resolution air pollution exposure prediction and spatiotemporal uncertainty characterization. This work requires significant computational resources due to the complexity and scale of the task. Specifically, the model predicts daily air pollutant concentrations (PM2.5 and NO2 at a 1 km grid resolution across the United States, spanning the years 2010–2018. Each daily prediction dataset is approximately 6 GB in size, resulting in substantial storage and processing demands.
To ensure efficient training, validation, and execution of the ensemble models at a national scale, I need access to GPU clusters with the following resources:
- Permanent storage: ≥100 TB
- Temporary storage: ≥50 TB
- RAM: ≥725 GB
In addition to MATLAB, I also require Python and R installed on the system. I use Python notebooks to analyze output data and run R packages through a conda environment in Jupyter Notebook. These tools are essential for post-processing and visualization of model predictions, as well as for running complementary statistical analyses.
To finalize the GPU system configuration based on my requirements and initial runs, I would appreciate guidance from an expert. Since I already have approval for the ACCESS Accelerate allocation, this support will help ensure a smooth setup and efficient utilization of the allocated resources.
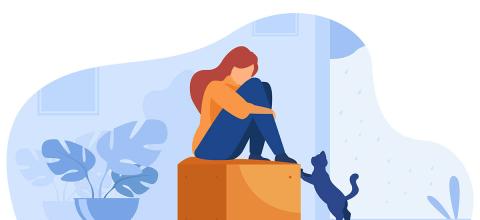
Investigation of robustness of state of the art methods for anxiety detection in real-world conditions
I am new to ACCESS. I have a little bit of past experience running code on NCSA's Blue Waters. As a self-taught programmer, it would be interesting to learn from an experienced mentor.
Here's an overview of my project:
Anxiety detection is topic that is actively studied but struggles to generalize and perform outside of controlled lab environments. I propose to critically analyze state of the art detection methods to quantitatively quantify failure modes of existing applied machine learning models and introduce methods to robustify real-world challenges. The aim is to start the study by performing sensitivity analysis of existing best-performing models, then testing existing hypothesis of real-world failure of these models. We predict that this will lead us to understand more deeply why models fail and use explainability to design better in-lab experimental protocols and machine learning models that can perform better in real-world scenarios. Findings will dictate future directions that may include improving personalized health detection, careful design of experimental protocols that empower transfer learning to expand on existing reach of anxiety detection models, use explainability techniques to inform better sensing methods and hardware, and other interesting future directions.