Affinity Groups
Logo | Name | Description | Tags | Join |
---|---|---|---|---|
AI Institutes Cyberinfrastructure | Gathering place for AI researchers to find curated information about using ACCESS resources for AI applications and research. | aimachine-learninggpu +1 more tags | Login to join |
Announcements
Title | Date |
---|---|
CI Pathways: Leading the Way to Effective CI Use | 02/20/25 |
Upcoming Events & Trainings
Topics from Ask.CI
Knowledge Base Resources
Title | Category | Tags | Skill Level |
---|---|---|---|
ACES: Charliecloud Containers for Scientific Workflows (Tutorial) | Learning | ACESTAMUSCRATCH +10 more tags | Beginner |
Advanced Mathematical Optimization Techniques | Learning | optimizationpython | Beginner, Intermediate, Advanced |
AHPCC documentary | Docs | loginbatch-jobsslurm +4 more tags | Beginner, Intermediate |
Engagements
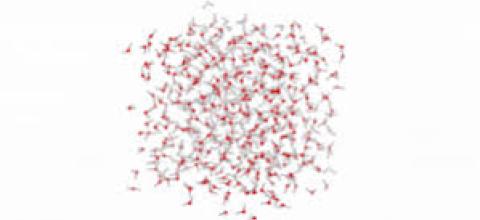
Re-engineering Lilly’s KisunlaTM into a novel antibody targeting IL13RA2 against GBM using AI-driven macromolecular modeling
- Summary and objectives of the proposed experiments:
- An initial research-based Ab (scFv47, discovered by our collaborator Dr. Balyasnikova) model, modeling Ab-Ag (IL13RA2 against GBM) protein complex, and identifying the binding sites (epitopes) using ROSETTA and AlphaFold2 multimer tools.
- Graft the CDRs of scFv (single-chain variable fragment) of antibody or Bispecific T cell engagers (BTEs) onto the template Ab, the framework of Lilly's Kisunla™ Ab drug.
- Modify, improve, and optimize the overall or full antibody protein structures using AI-driven macromolecule modeling (AlphaFold3).
- Explore single nucleotide polymorphism (SNP), pathogenic genetic variants and N-glycosylation of IL13RA2 (target) protein domain interacting with the Ab candidates among the patient population using ROSETTA software packages.
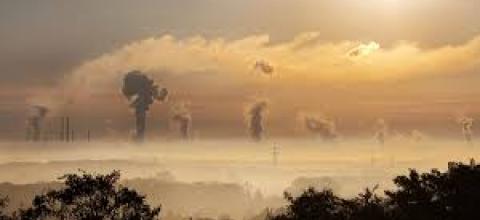
Bayesian nonparametric ensemble air quality model predictions at high spatio-temporal daily nationwide 1 km grid cell
I aim to run a Bayesian Nonparametric Ensemble (BNE) machine learning model implemented in MATLAB. Previously, I successfully tested the model on Columbia's HPC GPU cluster using SLURM. I have since enabled MATLAB parallel computing and enhanced my script with additional lines of code for optimized execution.
I want to leverage ACCESS Accelerate allocations to run this model at scale.
The BNE framework is an innovative ensemble modeling approach designed for high-resolution air pollution exposure prediction and spatiotemporal uncertainty characterization. This work requires significant computational resources due to the complexity and scale of the task. Specifically, the model predicts daily air pollutant concentrations (PM2.5 and NO2 at a 1 km grid resolution across the United States, spanning the years 2010–2018. Each daily prediction dataset is approximately 6 GB in size, resulting in substantial storage and processing demands.
To ensure efficient training, validation, and execution of the ensemble models at a national scale, I need access to GPU clusters with the following resources:
- Permanent storage: ≥100 TB
- Temporary storage: ≥50 TB
- RAM: ≥725 GB
In addition to MATLAB, I also require Python and R installed on the system. I use Python notebooks to analyze output data and run R packages through a conda environment in Jupyter Notebook. These tools are essential for post-processing and visualization of model predictions, as well as for running complementary statistical analyses.
To finalize the GPU system configuration based on my requirements and initial runs, I would appreciate guidance from an expert. Since I already have approval for the ACCESS Accelerate allocation, this support will help ensure a smooth setup and efficient utilization of the allocated resources.