Modelling conformational heterogeneity for human glutamine synthetase variants
<p>Hi!</p><p>I want to use <a href="https://github.com/COSBlab/EMMIVox">EMMIVox</a> molecular dynamics based ensemble refinement to model into multiple cryoEM maps that my lab has generated. I am a collaborator of Max Bonomi (PI behind EMMIVox) but also want to be able to do this independently. We have pre-processed single-refinement models but need multiple GPUs to run the ensemble refinement. I am new to NSF ACCESS and also new to ensemble refinement/molecular dynamics in general. Specifically, I need help to:</p><ul><li>Allocate Resources (which is best/how much is needed)</li><li>Software Installation (plumed dependencies and the software in the github link)</li><li>Job running/management </li></ul><p>I am running this part of the project myself and have not staffed this project yet with a student; so a mentor would be working directly with me. I would like assistance to run this procedure through at least once so that I can reach independence and start training my students to implement it.</p><p>Thank you so much in advance for any help and considering whether the MATCH+ program would be suitable for this project. </p><p>All the best,</p><p>Eric</p>
Re-engineering Lilly’s KisunlaTM into a novel antibody targeting IL13RA2 against GBM using AI-driven macromolecular modeling
<ul><li><strong>Summary and objectives of the proposed experiments: </strong></li></ul><ol><li>An initial research-based Ab (scFv47, discovered by our collaborator Dr. Balyasnikova) model, modeling Ab-Ag (IL13RA2 against GBM) protein complex, and identifying the binding sites (epitopes) using ROSETTA and AlphaFold2 multimer tools.</li><li>Graft the CDRs of scFv (single-chain variable fragment) of antibody or Bispecific T cell engagers (BTEs) onto the template Ab, the framework of Lilly's Kisunla™ Ab drug.</li><li>Modify, improve, and optimize the overall or full antibody protein structures using AI-driven macromolecule modeling (AlphaFold3).</li><li>Explore single nucleotide polymorphism (SNP), pathogenic genetic variants and <em>N</em>-glycosylation of IL13RA2 (target) protein domain interacting with the Ab candidates among the patient population using ROSETTA software packages.</li></ol>
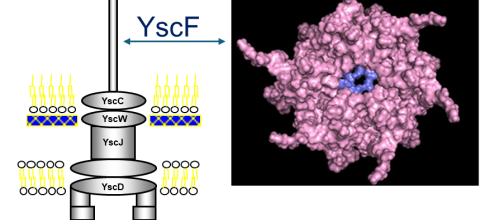
Prediction of Polymerization of the Yersinia Pestis Type III Secretion System
<p>Yersinia pestis, the bacterium that causes the bubonic plague, uses a type III secretion system (T3SS) to inject toxins into host cells. The structure of the Y. pestis T3SS needle has not been modeled using AI or cryo-EM. T3SS in homologous bacteria have been solved using cryo-EM. Previously, we created possible hexamers of the Y. pestis T3SS needle protein, YscF, using CollabFold and AlphaFold2 Colab on Google Colab in an effort to understand more about the needle structure and calcium regulation of secretion. Hexamers and mutated hexamers were designed using data from a wet lab experiment by Torruellas et. al (2005). T3SS structures in homologous organisms show a 22 or 23mer structure where the rings of hexamers interlocked in layers. When folding was attempted with more than six monomers, we observed larger single rings of monomers. This revealed the inaccuracies of these online systems. To create a more accurate complete needle structure, a different computer software capable of creating a helical polymerized needle is required. The number of atoms in the predicted final needle is very high and more than our computational infrastructure can handle. For that reason, we need the computational resources of a supercomputer. We have hypothesized two ways to direct the folding that have the potential to result in a more accurate needle structure. The first option involves fusing the current hexamer structure into one protein chain, so that the software recognizes the hexamer as one protein. This will make it easier to connect multiple hexamers together. Alternatively, or additionally the cryo-EM structures of the T3SS of Shigella flexneri and Salmonella enterica Typhimurium can be used as models to guide the construction of the Y. pestis T3SS needle. The full AlphaFold library or a program like RoseTTAFold could help us predict protein-protein interactions more accurately for large structures. Based on our needs we have identified the TAMU ACES, Rockfish and Stampede-2 as promising resources for this project. The generated model of the Y. pestis T3SS YscF needle will provide insight into a possible structure of the needle. </p>
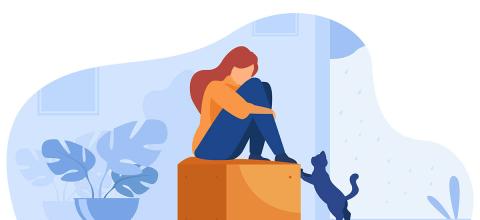
Investigation of robustness of state of the art methods for anxiety detection in real-world conditions
<p>I am new to ACCESS. I have a little bit of past experience running code on NCSA's Blue Waters. As a self-taught programmer, it would be interesting to learn from an experienced mentor. </p><p>Here's an overview of my project:</p><p>Anxiety detection is topic that is actively studied but struggles to generalize and perform outside of controlled lab environments. I propose to critically analyze state of the art detection methods to quantitatively quantify failure modes of existing applied machine learning models and introduce methods to robustify real-world challenges. The aim is to start the study by performing sensitivity analysis of existing best-performing models, then testing existing hypothesis of real-world failure of these models. We predict that this will lead us to understand more deeply why models fail and use explainability to design better in-lab experimental protocols and machine learning models that can perform better in real-world scenarios. Findings will dictate future directions that may include improving personalized health detection, careful design of experimental protocols that empower transfer learning to expand on existing reach of anxiety detection models, use explainability techniques to inform better sensing methods and hardware, and other interesting future directions.</p>
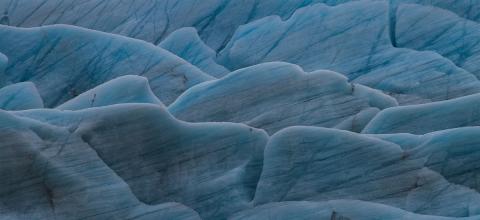
GPU-accelerated Ice Sheet Flow Modeling
<p>Sea levels are rising (3.7 mm/year and increasing!)! The primary contributor to rising sea levels is enhanced polar ice discharge due to climate change. However, their dynamic response to climate change remains a fundamental uncertainty in future projections. Computational cost limits the simulation time on which models can run to narrow the uncertainty in future sea level rise predictions. The project's overarching goal is to leverage GPU hardware capabilities to significantly alleviate the computational cost and narrow the uncertainty in future sea level rise predictions. Solving time-independent stress balance equations to predict ice velocity or flow is the most computationally expensive part of ice-sheet simulations in terms of computer memory and execution time. The PI developed a preliminary ice-sheet flow GPU implementation for real-world glaciers. This project aims to investigate the GPU implementation further, identify bottlenecks and implement changes to justify it in the price to performance metrics to a "standard" CPU implementation. In addition, develop a performance portable hardware (or architecture) agnostic implementation.</p>
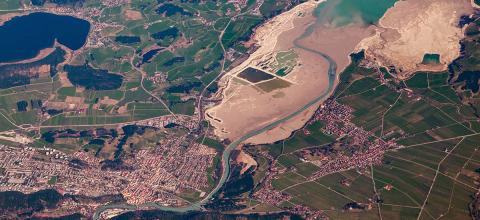
Adapting a GEOspatial Agent-based model for Covid Transmission (GeoACT) for general use
<p>GeoACT (GEOspatial Agent-based model for Covid Transmission) is a designed to simulate a range of intervention scenarios to help schools evaluate their COVID-19 plans to prevent super-spreader events and outbreaks. It consists of several modules, which compute infection risks in classrooms and on school buses, given specific classroom layouts, student population, and school activities. The first version of the model was deployed on the Expanse (and earlier, COMET) resource at SDSC and accessed via the Apache Airavata portal (geoact.org). The second version is a rewrite of the model which makes it easier to adjust to new strains, vaccines and boosters, and include detailed user-defined school schedules, school floor plans, and local community transmission rates. This version is nearing completion. We’ll use Expanse to run additional scenarios using the enhanced model and the newly added meta-analysis module. The current goal is to make the model more general so that it can be used for other health emergencies. GeoACT has been in the news, e.g. <a href="https://ucsdnews.ucsd.edu/feature/uc-san-diego-data-science-undergrads-… San Diego Data Science Undergrads Help Keep K-12 Students COVID-Safe</a>, and <a href="https://www.hpcwire.com/2022/01/13/sdsc-supercomputers-helped-enable-sa… Supercomputers Helped Enable Safer School Reopenings</a> (HPCWire 2022 Editors' Choice Award)</p>
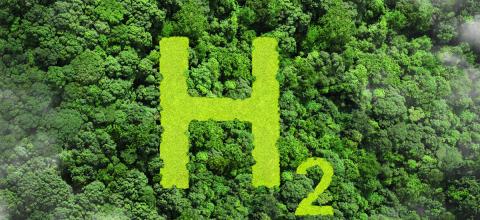
Exploring Small Metal Doped Magnesium Hydride Clusters for Hydrogen Storage Materials
<p>Solid metal hydrides are an attractive candidates for hydrogen storage materials. Magnesium has the benefit of being inexpensive, abundant, and non-toxic. However, the application of magnesium hydrides is limited by the hydrogen sorption kinetics. Doping magnesium hydrides with transition metal atoms improves this downfall, but much is still unknown about the process or the best choice of dopant type and concentration.</p><p>In this position, the student will study magnesium hydride clusters doped with early transition metals (e.g., Ti and V) as model systems for real world hydrogen storage materials. Specifically, we will search each cluster's potential energy surface for local and global minima and explore the relationship of cluster size and dopant concentration on different properties. The results from this investigation will then be compared with related cluster systems.</p><p>The student will begin by performing a literature search for this system, which will allow the student to pick an appropriate level of theory to conduct this investigation. This level will be chosen by performing calculations on the MgM, MgH, and MH (M = Ti and V) diatomic species (and select other sizes based on the results of the literature search) and comparing the predictions with experimentally determined spectroscopic data (e.g., bond length, stretching frequency, etc.). The student will then perform theoretical chemistry calculations using the Gaussian 16 and NBO 7 programs on the EXPANSE cluster housed at the San Diego Supercomputing Center (SDSC) through ACCESS allocation CHE-130094. First, this student will generate candidate structures for each cluster size and composition using two global optimization procedures. One program utilizes the artificial bee colony algorithm, whereas the second basin hoping program is written and compiled in-house using Fortran code. Additional structures will be generated by hand from our prior knowledge. All candidate structures will then be further optimized by the student at the appropriate level determined at the start of the semester. Higher level (e.g., double hybrid density functional theory) calculations will also be performed as further confirmation of the predicted results. Various results will be visualized with the Avogadro, Gabedit, and Gaussview programs on local machines. </p>
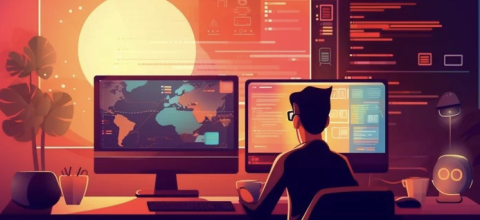
AI for Business
<p>The research focus is to apply the pre-training techniques of Large Language Models to the encoding process of the Code Search Project, to improve the existing model and develop a new code searching model. The assistant shall explore a transformer or equivalent model (such as GPT-3.5) with fine-tuning, which can help achieve state-of-the-art performance for NLP tasks. The research also aims to test and evaluate various state-of-the-art models to find the most promising ones.</p>
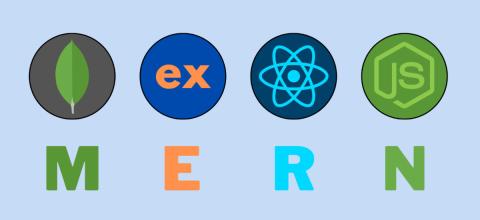
Web Deployment for Undergraduates
<p>Issue: I am teaching a web development course (MERN stack) and do not have a deployment set up. I would like to use Jetstream2 because they offer instances with IP addresses. I have enough allocations for 20 students to each have their own instance. I was able to build a MERN stack and deploy a static react site, but the backend MongoDB piece, while connected, did not serve up data. I cannot figure out why. Helpdesk at Jetstream suggest trying a docker container, which makes sense but obviously if I can’t get it working one way I’ll need help.</p>
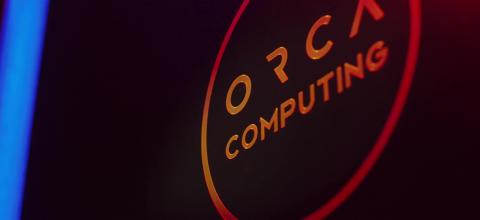
CHE230120: Natural Bond Order Calculation of Fe(II) isonitrile complex using the ORCA computational suite
<p>I am self-taught on how to use the ORCA computational suite on my university supercomputer cluster via microsoft visual studio code. Problem is that we don't have the natural bond order software package for ORCA, so I can't do a specific kind of calculation (NBO calc).</p><p>I have an ACCESS grant to do these calculations, and I'm looking for a generous soul to shepard me through my first calculation using this resource.</p><p>I need to:</p><ol><li>Identify the appropriate resource (from my research it seems the SDSC expanse cluster has ORCA...not sure if the NBO suite is installed but I'd be surprised if it wasn't at a well-off place like UCSD )</li><li>Learn how to gain access / log into / Navigate the computer resource</li><li>Prepare a jobscript to submit an ORCA computational job (I know how to prepare one for my Uni's cluster, but I'm not a computer sci person)</li><li>access, download, save the resulting computations</li></ol><p> </p>