Self-gravity and Contact detection Optimisation in LMGC90
This project concerns that parallelisation and optimisation of LMGC90. LMGC90 is an open-source, multi-purpose Physics code developed at the University of Montpellier (UofM). In the last few years, we have used this code to simulate self-gravitating granular systems, which in turn have been used as proxies for small asteroids and other small Solar System bodies [1][2]. To do this, the code uses an external, open-source python library (pykdgrav) to calculate self-gravity between the simulated particles. This library uses and kd-tree and has been parallelised by its authors.
LMGC90 [3] has a contact detection algorithm of its own, but this is neither parallelised nor coupled to pykdgrav [4]. So, at the moment, when the code is used to simulate self-gravitating systems, distances are calculated twice.
The objective of the project is to fully integrate pykdgrav withing LMGC90, possibly use it as or as part of the contact detection algorithm and so have this part of the code completely parallelised and optimised to simulate self-gravitating granular systems. If the self-gravity and contact detection algorithm are kept separated, the former should still be fully integrated into LMGC90 and the latter should be parallelised.
At the moment, there are only a handful of simulation codes that can handle self-gravitating granular systems that can be used by the scientific community at large and not all of them are as mature as LMGC90. Additionally, NASA's latest space missions to asteroids and their space exploration and Planetary Sciences objectives require this kind of simulation to be reached. We expect this code to be used to be used as a tool in this endeavour.
The student working with us will be mentored by the developers of the code (UofM) and users of the code who work in the project at UofM and the University of Colorado Boulder.
ACCESS resources that would be needed are unknown at the moment.
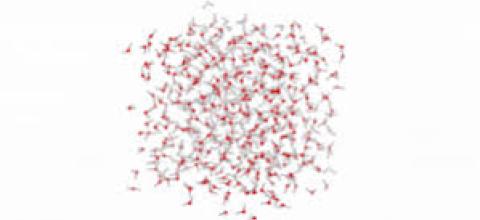
Re-engineering Lilly’s KisunlaTM into a novel antibody targeting IL13RA2 against GBM using AI-driven macromolecular modeling
- Summary and objectives of the proposed experiments:
- An initial research-based Ab (scFv47, discovered by our collaborator Dr. Balyasnikova) model, modeling Ab-Ag (IL13RA2 against GBM) protein complex, and identifying the binding sites (epitopes) using ROSETTA and AlphaFold2 multimer tools.
- Graft the CDRs of scFv (single-chain variable fragment) of antibody or Bispecific T cell engagers (BTEs) onto the template Ab, the framework of Lilly's Kisunla™ Ab drug.
- Modify, improve, and optimize the overall or full antibody protein structures using AI-driven macromolecule modeling (AlphaFold3).
- Explore single nucleotide polymorphism (SNP), pathogenic genetic variants and N-glycosylation of IL13RA2 (target) protein domain interacting with the Ab candidates among the patient population using ROSETTA software packages.
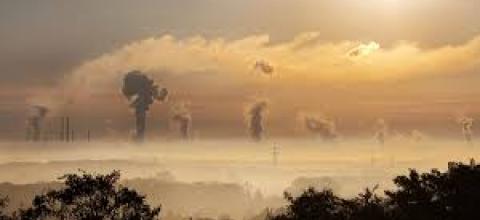
Bayesian nonparametric ensemble air quality model predictions at high spatio-temporal daily nationwide 1 km grid cell
I aim to run a Bayesian Nonparametric Ensemble (BNE) machine learning model implemented in MATLAB. Previously, I successfully tested the model on Columbia's HPC GPU cluster using SLURM. I have since enabled MATLAB parallel computing and enhanced my script with additional lines of code for optimized execution.
I want to leverage ACCESS Accelerate allocations to run this model at scale.
The BNE framework is an innovative ensemble modeling approach designed for high-resolution air pollution exposure prediction and spatiotemporal uncertainty characterization. This work requires significant computational resources due to the complexity and scale of the task. Specifically, the model predicts daily air pollutant concentrations (PM2.5 and NO2 at a 1 km grid resolution across the United States, spanning the years 2010–2018. Each daily prediction dataset is approximately 6 GB in size, resulting in substantial storage and processing demands.
To ensure efficient training, validation, and execution of the ensemble models at a national scale, I need access to GPU clusters with the following resources:
- Permanent storage: ≥100 TB
- Temporary storage: ≥50 TB
- RAM: ≥725 GB
In addition to MATLAB, I also require Python and R installed on the system. I use Python notebooks to analyze output data and run R packages through a conda environment in Jupyter Notebook. These tools are essential for post-processing and visualization of model predictions, as well as for running complementary statistical analyses.
To finalize the GPU system configuration based on my requirements and initial runs, I would appreciate guidance from an expert. Since I already have approval for the ACCESS Accelerate allocation, this support will help ensure a smooth setup and efficient utilization of the allocated resources.
Study of Phase Transition in Two-Photon Dicke Model
I am not familiar with exchanging credits for hours on machine and any guidance as which cluster and how many hours needed on it would be great. I am experienced in python coding and would like to work on a python friendly interface and i need to parallelize my code to run for lots of different parameter values and it also involves large matrix so quite some memory overhead.